Driving Proactive IT Excellence: Transforming Operations with AI/ML-Enhanced CMDB
The implementation of an AI/ML-enhanced CMDB revolutionized the IT Operations
- Home
- Case Studies
- Driving Proactive IT Excellence: Transforming Operations with AI/ML-Enhanced CMDB
Executive Summary
A German automobile manufacturing company with a vast and intricate IT environment struggled to maintain an accurate and up-to-date Configuration Management Database (CMDB). Manual data entry and frequent changes made it challenging to track assets, dependencies, and relationships accurately.
Solution
Crest implemented an AI/ML-enhanced CMDB solution to address these challenges:
- Data Integration: Data from various sources, including discovery tools, monitoring systems, and manual input were integrated into a centralized CMDB. The CMDB ingested terabytes of data from multiple data sources, including hardware and software inventories, network traffic, user activity logs, and application performance metrics.
- Data Enrichment: The raw data was enriched with additional information, such as usage patterns, historical changes, and interdependencies between assets.
Enrichment included data from previous maintenance logs, software upgrade histories, and user access patterns.
- AI-Powered Discovery and Classification:
Deep Learning-based Natural Language Processing (NLP) algorithms were used for automated discovery of new assets and to identify patterns in data that required categorization.
Unsupervised learning techniques, such as clustering, were applied to group assets based on common attributes and usage profiles.
- Dependency Mapping: Graph-based Machine Learning models were employed to map complex relationships and dependencies among different assets, applications, and services.
Graph algorithms like PageRank and community detection were used to identify critical nodes, bottlenecks, and cascading effects.
- Anomaly Detection:
Time Series Analysis models, such as AutoRegressive Integrated Moving Average (ARIMA), were used for detecting unusual changes in the CMDB over time.
Any deviations from historical usage and configuration patterns triggered alerts for further investigation.
- Predictive Insights:
Regression models, such as Random Forest or Gradient Boosting, were trained to predict potential issues based on historical data.
Features used for prediction included historical workload trends, resource utilization, and patterns of configuration changes.
Results
The AI/ML-enhanced CMDB solution delivered several positive outcomes:
- Automation reduced manual data entry errors, ensuring the CMDB remained accurate and up-to-date in real time.
- The system automatically identified and tracked configuration changes, streamlining the change management process.
- Predictive insights allowed the IT team to take preemptive actions, reducing incidents and minimizing downtime.
- Dependency mapping and usage insights enabled better allocation of resources, enhancing performance and cost-effectiveness.
- Automation and AI-driven discovery saved significant time that was previously spent on manual data collection and validation.
- Accurate and auditable CMDB data supported compliance efforts by providing a reliable record of the IT environment.
Conclusion
The implementation of an AI/ML-enhanced CMDB revolutionized the IT Operations. By automating data entry, enhancing accuracy, and providing predictive insights, the company achieved greater efficiency, reduced downtime, and improved overall IT service delivery. This case study demonstrates the transformative power of AI and ML in enhancing the capabilities of CMDB for proactive IT Operations.
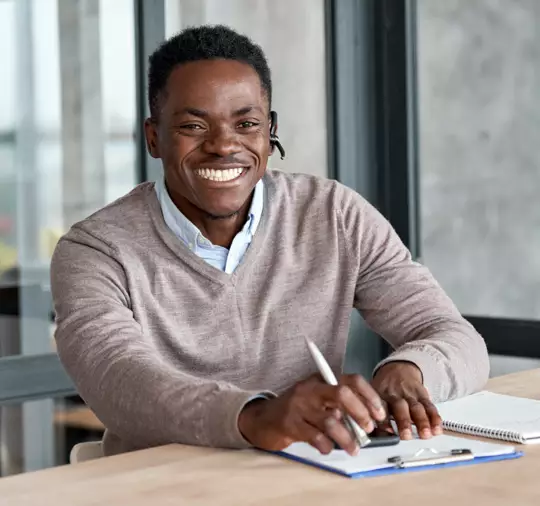